Define and Execute New Benchmark for Machine Learning Use Case in Finance
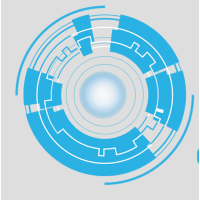
Project scope
Categories
Data analysis Market research Information technologySkills
software systems financial controls internal controls anti money laundering business metrics openroads (civil design software) invoicing business valuation fraud detection machine learningProblem Background:
AI and machine learning are making more inroads into the financial services industry. Beyond the established use of AI for fraud detection and anti-money laundering, the use of AI and ML continues to push into other business cases, including the use for bank back office processes such as transaction reconciliations used for financial control and reporting. For example, those performed for matching bank transactions to business activities such as expenses, invoices or trading.
One of the difficulties that customers have in evaluating such ML based products is that the business value of these products is defined by the efficacy and accuracy of the ML model rather than being based on the particular product features (as it is for standard procedural software systems).
Traditional research / product advisory firms such as Gartner, Celent, EY, Accenture etc create feature based vendor product evaluation reports, but none as yet provide any quantitative measurement of the ML functionality in vendor products.
Some existing benchmark projects:
- One organization in this area is starting to look into industry ML benchmarks is STAC.com ( https://www.enterpriseai.news/2019/01/16/stac-floats-ml-benchmark-for-financial-services-workloads/ ). However they are currently focussed more on measuring model processing speed rather than model effectiveness. They also do not have any benchmarks for transaction matching.
- The https://mlperf.org/ initiative is another recently setup set of non industry specific ML based benchmarks, but again these are focussed on the processing speed of the model rather than the efficacy. They also do not have any benchmarks for transaction matching.
- Kaggle.com has ML competitions for some financial data sets, but does not have any for transaction matching.
This project aims to setup the first industry benchmark for this ubiquitous business problem.
Project Goal:
- Design a set of benchmarks which capture the key business metrics for this problem, (this may involve interviewing customers whose pain point is being addressed, contacts will be provided). Also, discussion with advisory firms to help define and setup benchmarks in this field.
- Contact leading vendors for reconciliation engines (Operartis will provide contact details for vendors)
- Create a consortium of the leading vendors to help design the different data sets and benchmarks.
- Design, generate and gather an appropriate set of training and evaluation data sets (see below for some possible sources)
- Evaluate the market leading reconciliation engines on these data sets.
- Generate a report on the findings.
Data set sources include:
- Operartis example data sets.
- Simulated data generation (Operartis has an existing data simulator which can be used to generate a variety of test data sets.)
- Other vendor data sets.
- https://fintechsandbox.org Real world financial data sets
- Internal organization data sets (e.g. from your university)
You will work with the core Operartis team and meet weekly with the CEO as the project progresses and will be able to adapt, augment this general plan as the project progresses. The Operartis team is a compact, friendly team where you will have a welcoming place to express your creativity and problem solving skills.
About the company
Founded in New York by veterans of the banking industry our mission is to provide machine learning-based technology solutions that push automation and straight-through processing to the next level, driving down the need for tedious manual work and freeing up the most precious resource an organization has: its people.