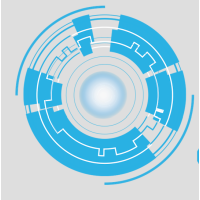
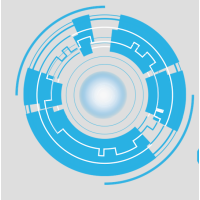
Virtual Internship Opportunity: Computer Science
Positions available: 1 team of 2 person Our organization is able to offer a virtual internship opportunity for computer science students or teams. The primary focus for the student will be: (please include a few examples of technology projects you would like the student(s) to execute during the placement, as exemplified below. Where applicable, please indicate tools/platforms the student(s will be using to complete their work for you.) Operartis is deploying its machine learning java and Kotlin based solution to the cloud-based financial services platform https://www.fusionfabric.cloud . We are seeking students to assist with the end to end API build, Finastra validation process and launch on the Finastra app store. Our goal at the end of this experience is: For the student(s) to create a fully functioning API for Matchimus into the Finastra cloud environment and to see this application through the official Finastra validation process, document the API and testing and assist in the launch into the application store by a deadline of May 31st 2021.
Marketing Intern
Positions Available: 1 team of 2 person We have a social media strategy plan which we need to implement. We’re looking for a student to help us source, curate, develop, and post the content generation processes to increase awareness and nurture engagement. In particular we have new machine learning benchmark initiative which we need to promote. Based on our goals, we are looking for the student to work on the following: Fun and engaging linked in Content Calendar Curation: timely deployment of all our social media content to drive maximum traffic to our site Social Media Management: overseeing different channels that we own and keeping them up-to-date Content Development with input from sales team. SEO Other marketing-related tasks such as newsletters, case studies and press releases.
Execute first benchmarks on transaction matching for financial institutions
ABOUT COMPANY Founded in New York by veterans of the banking industry our mission is to provide machine learning-based technology solutions that push automation and straight-through processing to the next level, driving down the need for tedious manual work and freeing up the most precious resource an organization has: its people. Our flagship product is our groundbreaking machine learning based match rate booster for reducing the manual effort needed for transaction reconciliations. PROJECT SCOPE Problem Background: AI and machine learning are making more inroads into the financial services industry. Beyond the established use of AI for fraud detection and anti-money laundering, the use of AI and ML continues to push into other business cases, including the use for bank back office processes such as transaction reconciliations used for financial control and reporting. For example, those performed for matching bank transactions to business activities such as expenses, invoices or trading. One of the difficulties that customers have in evaluating such ML based products is that the business value of these products is defined by the efficacy and accuracy of the ML model rather than being based on the particular product features (as it is for standard procedural software systems). Traditional research / product advisory firms such as Gartner, Celent, EY, Accenture etc create feature based vendor product evaluation reports, but none as yet provide any quantitative measurement of the ML functionality in vendor products. This project aims to setup the first industry benchmark for this ubiquitous business problem. Project Goal: Take existing Operartis data sets and clean and anonymize these for external use. Formally document these datasets such that external parties can utilize the datasets and set them up in their reconciliation engines. Create tools to show the match rate, accuracy and reliability curves and design other repeatable metrics in line with benchmark best practices. Optional additional goals: Collaborate with advisory firms to help execute the benchmarks for different customers. Assist with outreach to leading vendors for reconciliation engines (Operartis will provide contact details for vendors) (Optional) Create a consortium of the leading vendors to help design the different data sets and benchmarks. Where possible evaluate the market leading reconciliation engines on these data sets. Generate a report on the findings. Data set sources include: Operartis example data sets. Simulated data generation (Operartis has an existing data simulator which can be used to generate a variety of test data sets.) Other vendor data sets. https://fintechsandbox.org Real world financial data sets Internal organization data sets (e.g. from your university) You will work with the core Operartis team and meet weekly with the CEO as the project progresses and will be able to adapt, augment this general plan as the project progresses. The Operartis team is a compact, friendly team where you will have a welcoming place to express your creativity and problem solving skills.
INSIGHT-SELLING AND PARTNERSHIP STRATEGY.2
ABOUT COMPANY Founded in New York by veterans of the banking industry our mission is to provide machine learning-based technology solutions that push automation and straight-through processing to the next level, driving down the need for tedious manual work and freeing up the most precious resource an organization has: its people. PROJECT SCOPE Our organization has developed a novel machine learning-based solution to a pain point for financial institutions during transaction reconciliation processes. (The most prevalent and most widely known example of a transaction reconciliation is a cash reconciliation which is performed between business activity transactions such as expenses, invoices, trades etc and the associated cash movements.) We would like to improve/develop the following in order to grow our sales: Prospecting Strategy - Evaluate our current leads base and make recommendations for further market penetration; assess our current outreach process and develop and implement targeted prospecting; review our prospect messaging, make improvement recommendations, and create new outreach content. Review and improve our existing sales process and sales playbook. We recently developed the industry's first survey/assessment which focuses on and quantifies this industry pain-point and would like to use this survey and it’s finding to create an insights-driven sales process . We also just launched datasets for use for benchmarking of machine learning matching solutions which we would like to use incorporate into our sales process a scientific proof-driven style of selling . Develop channel partner, affiliate marketing and platform as a service strategy. We already established a partnership with one of the Big 5 consulting firms and would like to continue building out relationships with other partners. We would like to develop a well-constructed, attractive and standardized value proposition, referral program, revenue share model etc, co-branding. You will work with the core Operartis team and meet as frequently you need to with the CEO as the project progresses. The Operartis team is a compact, friendly team where you will have a welcoming place to express your creativity and problem solving skills.
INSIGHT-SELLING AND PARTNERSHIP STRATEGY
ABOUT COMPANY Founded in New York by veterans of the banking industry our mission is to provide machine learning-based technology solutions that push automation and straight-through processing to the next level, driving down the need for tedious manual work and freeing up the most precious resource an organization has: its people. PROJECT SCOPE Our organization has developed a novel machine learning-based solution to a pain point for financial institutions during transaction reconciliation processes. (The most prevalent and most widely known example of a transaction reconciliation is a cash reconciliation which is performed between business activity transactions such as expenses, invoices, trades etc and the associated cash movements.) We would like to improve/develop the following in order to grow our sales: Prospecting Strategy - Evaluate our current leads base and make recommendations for further market penetration; assess our current outreach process and develop and implement targeted prospecting; review our prospect messaging, make improvement recommendations, and create new outreach content. Review and improve our existing sales process and sales playbook. We recently developed the industry's first survey/assessment which focuses on and quantifies this industry pain-point and would like to use this survey and it’s finding to create an insights-driven sales process . We also just launched datasets for use for benchmarking of machine learning matching solutions which we would like to use incorporate into our sales process a scientific proof-driven style of selling . Develop channel partner, affiliate marketing and platform as a service strategy. We already established a partnership with one of the Big 5 consulting firms and would like to continue building out relationships with other partners. We would like to develop a well-constructed, attractive and standardized value proposition, referral program, revenue share model etc, co-branding. You will work with the core Operartis team and meet as frequently you need to with the CEO as the project progresses. The Operartis team is a compact, friendly team where you will have a welcoming place to express your creativity and problem solving skills.
SEO Strategy, Content calendar
We would like to drive more traffic to our website. We would like to be on the first page of google for manual transaction questions. We know that we need to implement SEO, and we have improved some of our keyword searches but are still not ranking highly on some key terms. We would like to collaborate with students to understand what we need to do to generate more traffic and revenue through search engines. Complete an SEO analysis on our website Conduct competitive keyword research Update our website with the best structure and elements for SEO. Develop an on-page and off-page SEO plan. Identify key metrics to track for success. Analyse visitor characteristics, make suggestions to improve visitor engagement and click through Planning a content calendar to drive traffic to our site. Placement of surveys.
Digital Marketing Strategy and Development
Operartis is a B2B, machine-learning startup looking for virtual internship for (1 | 2 ) ambitious and creative marketing students to help position Operartis’ disruptive technology in the existing competitive landscape. The primary focus for the intern(s) will be developing and beginning execution of a digital marketing strategy which will address the following key points: Competitive Analysis and Branding - Conduct a full competitive landscape assessment; define our organization's brand and core value proposition; identify opportunities and threats facing our brand; assess where and how to improve our brand's presence and differentiate from our competitors. Develop thought leadership education based marketing strategy with the design of educational seminars/webinars which offer concrete, useful information for our target audience. Topics to be covered can include using artificial intelligence in the finance industry, measuring AI ROI. Tips on how to run a successful efficiencies project etc. The five mistakes that managers make when implementing AI etc. Include insights based on interviews with key people in the industry. Validate the pricing strategy; Pricing strategy versus other vendor products, ROI etc. Develop channel partner and platform as a service strategy We are establishing a partnership with one of the Big 5 consulting firms and would like to continue building out relationships with other partners. Public Relations - Develop and implement a PR strategy which demonstrates thought leadership ; create collateral, including press releases, user testimonials, case studies, and customer stories. Prospecting Strategy - Evaluate our current leads base and make recommendations for further market penetration; assess our current outreach process and develop and implement targeted prospecting; review our prospect messaging, make improvement recommendations, and create new outreach content. SEO Strategy & Recommendatio ns - Develop and implement an SEO strategy; provide content suggestions and recommendations to increase search visibility; increase our inbound traffic and optimize the customer journey; increase inbound leads. Strategic Social Media Marketing - Conduct an audit of our current social media presence and/or other current communication channel(s); develop and implement strategies for improving our online presence and increasing engagement; create a content calendar; creating useable linkedin and blog posts. Our goal at the end of this experience is For each of the above strategies, document the strategy and implementable execution processes (in the form of handbooks and checklists) and perform initial execution of the strategy. This will include assisting with outbound email marketing campaigns, linked in posts; measure and report on performance of these campaigns. Content development, including LinkedIn articles, webinars, case studies and website copy. You will work with the core Operartis team and meet weekly with the CEO as the project progresses and will be able to adapt, augment this general plan as the project progresses. The Operartis team is a compact, friendly team where you will have a welcoming place to express your creativity and problem solving skills.
Define and Execute New Benchmark for Machine Learning Use Case in Finance
Problem Background: AI and machine learning are making more inroads into the financial services industry. Beyond the established use of AI for fraud detection and anti-money laundering, the use of AI and ML continues to push into other business cases, including the use for bank back office processes such as transaction reconciliations used for financial control and reporting. For example, those performed for matching bank transactions to business activities such as expenses, invoices or trading. One of the difficulties that customers have in evaluating such ML based products is that the business value of these products is defined by the efficacy and accuracy of the ML model rather than being based on the particular product features (as it is for standard procedural software systems). Traditional research / product advisory firms such as Gartner, Celent, EY, Accenture etc create feature based vendor product evaluation reports, but none as yet provide any quantitative measurement of the ML functionality in vendor products. Some existing benchmark projects: One organization in this area is starting to look into industry ML benchmarks is STAC.com ( https://www.enterpriseai.news/2019/01/16/stac-floats-ml-benchmark-for-financial-services-workloads/ ). However they are currently focussed more on measuring model processing speed rather than model effectiveness. They also do not have any benchmarks for transaction matching. The https://mlperf.org/ initiative is another recently setup set of non industry specific ML based benchmarks, but again these are focussed on the processing speed of the model rather than the efficacy. They also do not have any benchmarks for transaction matching. Kaggle.com has ML competitions for some financial data sets, but does not have any for transaction matching. This project aims to setup the first industry benchmark for this ubiquitous business problem. Project Goal: Design a set of benchmarks which capture the key business metrics for this problem, (this may involve interviewing customers whose pain point is being addressed, contacts will be provided). Also, discussion with advisory firms to help define and setup benchmarks in this field. Contact leading vendors for reconciliation engines (Operartis will provide contact details for vendors) Create a consortium of the leading vendors to help design the different data sets and benchmarks. Design, generate and gather an appropriate set of training and evaluation data sets (see below for some possible sources) Evaluate the market leading reconciliation engines on these data sets. Generate a report on the findings. Data set sources include: Operartis example data sets. Simulated data generation (Operartis has an existing data simulator which can be used to generate a variety of test data sets.) Other vendor data sets. https://fintechsandbox.org Real world financial data sets Internal organization data sets (e.g. from your university) You will work with the core Operartis team and meet weekly with the CEO as the project progresses and will be able to adapt, augment this general plan as the project progresses. The Operartis team is a compact, friendly team where you will have a welcoming place to express your creativity and problem solving skills.
Measuring the magnitude of a ubiquitous industry problem
Our organization has developed a novel solution to a ubiquitous industry pain point, but up to now this pain point has remained largely hidden , been identified only anecdotally and has never been formally analyzed, quantified and documented.... until of course you work on it!. The aim of this project is to perform an industry survey to rectify this situation and to produce the first published survey and analysis on this subject . The ubiquitous industry pain point relates to the following business process which is performed by every single organization in every single industry, but which is a particular pain point for financial institutions due to their high transaction volume. The problem occurs during transaction reconciliation processes. The most prevalent and most widely known example of a transaction reconciliation is a cash reconciliation which is performed between business activity transactions such as expenses, invoices, trades etc and the associated cash movements. To match these transactions, most organizations use either spreadsheet, or a purpose made 'rules based' matching engine. This approach works well when the data has high quality, but when corresponding transactions do not have exactly matching attributes, or some of the attributes are blank, or there are multiple transactions for similar amounts, this simple rules based approach does not deliver 100% matching or can sometimes mismatch transactions. In such situations an automated match rate may only be 80% which with 10's of millions of transactions a year can lead to millions of unmatched transactions which have to be matched manually by the organizations staff. This is tedious, time consuming work which not only costs time and effort, but also leads to delays in completing the transaction reconciliation. This project is to analyze, quantify and document the scale and business impact of this problem in the financial services industry. Specifically some of the details which this market/industry research should address are Determine across industry estimates of the volume of manual matching and mismatches. (Stratified by type of financial institution) How many people in each organization are working on this function? How much time does a manual match typically take for an operation staff? Has this ever caused a delay in close? In the light of Covid-19, is there a directive to reduce dependency on labor for this kinds of functions? What is the accounting impact / operational risk of transaction mismatches? Estimate the cost of the manual effort across the industry and from this the total addressable market size. We believe that there is enough industry data available which when combined with surveys/interviews will provide answers to these questions. We can provide appropriate industry contacts. Using these findings, we would like a detailed research report that will be a valuable resource for our customer and will help us to position ourselves as thought-leaders for this particular problem (and more generally for the use of machine learning to help automate back-office repetitive business processes in such a way that guarantees a stellar return on investment for the customer.) We believe this project will include but is not limited to: 1. Creating a survey and interviewing both owners and line workers who are focussed on the reconciliation function 2. Determining industry known volumes such as total payments moving through the Federal Reserve to provide an estimate for the magnitude of this issue. 3. Using the timing estimate gathered from interviewees/survey participants, determining the $ cost of this business problem. You will work with the core Operartis team and meet weekly with the CEO as the project progresses and will be able to adapt, augment this general plan as the project progresses. The Operartis team is a compact, friendly team where you will have a welcoming place to express your creativity and problem solving skills.